What is causal impact and why is it needed in a post-IDFA world?
August 09, 2021
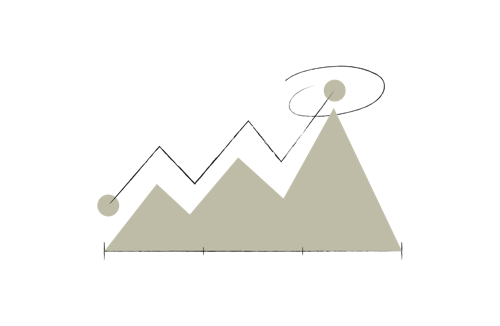
This article was inspired by a recent episode from Remerge’s Apptivate podcast, featuring Alicia Horsch, Data Scientist in the marketing analytics team at Socialpoint. Socialpoint is a game developer and publisher based in Barcelona and has been connecting the world through games since 2008.
You can listen to episode 95 of the Apptivate podcast through this link.
Until recently, marketers were able to track everything that any particular customer does online. Every customer journey was measured from start to end and generated millions of data points that provided insights on where to optimize marketing efforts. Apple’s iOS 14.5 and following versions have since deprecated the user ID or the Identifier for Advertisers (IDFA), due to the rising focus on user privacy. Without the IDFA, marketing activities can no longer be measured with the traditional attribution models.
Causal impact helps marketers understand how much of their installs and conversions actually belong to a marketing campaign. Since this methodology makes predictions based on real data that is coupled with a time-series component, hard-to-track marketing activities like TV campaigns and product launches that also have an effect on installs and conversions can also be accounted for.
What is Causal Impact Analysis?
In incrementality testing, Causal Impact is a methodology that is used to estimate the causal effect of no-ID marketing campaigns. The causal effect, unlike correlation, proves that something has happened or is happening because of something else. In mobile marketing, causal impact determines whether the ad caused a change in behavior such as when a user installs the app or purchases something because of it.
Causal Impact is a data library that provides the opportunity to optimize one's marketing portfolio based on recorded historical information. Using the Bayesian structural time-series (BSTS) technique, this statistical model provides a definitive way to measure marketing activities, contrary to probabilistic attribution models.
« We used to be able to track everyone and now we can’t do that anymore. That’s what makes this method really great for us. »
Alicia Horsch, Data Scientist, Socialpoint
Applying Causal Impact on Online Marketing Campaigns
Causal impact analysis works by using historical data to make a prediction on what would have happened if there would not have been a marketing campaign. This is often called a counterfactual, which is a statement of something that has not happened.
As with any test, the audience is divided into two groups. These groups are called the treatment group and the control group. During the incrementality test, ads are delivered to the treatment group, but not to the control group (it remains unexposed to ads).
Dividing the audience has become more challenging since the rollout of iOS 14.5, as randomization is no longer possible. Without the IDFA, marketers are coming up with new ways of splitting their audiences. Geographies, platforms, OS versions or device types, are some of the many ways of splitting audiences. It’s important to note that some parameters have their caveats. When targeting users based on different geos (i.e. Berlin vs. Hamburg), users can be in different places on the same day, consequently affecting the group split. Using device models is currently the most reliable way to split audiences.
In causal impact analysis, the treatment group behavior is predicted based on a control group. The uplift is defined as the difference between the actual and predicted counterfactual behavior seen in a specific time period.Treatment group KPIs like clicks or impressions are coupled with one or more covariates, oftentimes the control group, to measure the impact of the ads while a campaign is running.
For example, a campaign is running during a public holiday and installs go up. The covariate captures and accounts for this information and predicts the treatment group behavior within the campaign period. Another example can be seen in increased conversions on Sundays. With causal impact, the control group captures the trend and accounts for the difference. Any change that can’t be explained is measured as uplift.
In both scenarios, it’s important to make sure that only one experiment is carried out each time, as running multiple campaigns at the same time can affect the accuracy of the prediction.
Key Takeaway
After the launch of Apple’s App Tracking Transparency (ATT) framework, user-level tracking has become more limited, making marketing activities harder to measure and optimize. Using causal impact on incrementality testing allows marketers to assess the value of their campaigns through the use of historical data and predictive analytics.