Ep. 99 Data Science: Advanced Modeling for Mobile
August 27, 2021
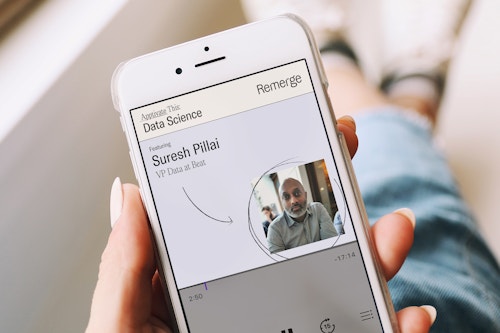
Today on our Data Science Segment, host Johannes Haupt interviews a theoretical physicist who sheds his expertise in complexity science, or complex systems, in the world of mobile apps. They discuss uplift modeling, incrementality and causal modeling using machine learning, multi-touch attribution, and marketing mix models.
Suresh Pillai is a theoretical physicist by training and the Vice President of Data at Beat. Beat is a technology company and ride-hailing app. Beat claims to be the fastest growing ride-hailing app in Latin America. The app provides an affordable, convenient, efficient and safe transportation option in modern megacities, aiming to improve the transportation infrastructure, while providing enormous economic opportunities to thousands of people who wish to earn income as drivers (p.s. they’re hiring).
Listen and Subscribe
Questions Suresh Answers In This Episode
- How do you approach mobile data science from your theoretical physics perspective?
- How have you used uplift modeling or incrementality?
- Define propensity in the context of uplift modeling.
- Can you explain in more detail the marketing settings you never turn off?
- What is the difference between how people use uplift modeling, incrementality and other causal machine learning?
- Do you have any tips for people to make sense of attribution in the complex setting of multi-touch marketing?
- We’re losing unique identifiers for users with the change to iOS14. What does this change for you? Has it been a problem? And do you think there’s a role for marketing mix models here?
- What’s the most interesting insights you’ve seen from incrementality models? What really surprised you? What changed your view on how customers are acting?
Timestamp
- 0:41 Suresh’s background & complexity science
- 2:14 A physicist’s view of complex systems in mobile data science
- 5:03 The granularity of incrementality and uplift modeling
- 6:05 Sure things, persuadables, lost causes, and sleeping dogs
- 11:31 Uplift modeling when there is no baseline
- 13:31 Uplift vs causal vs attribution models
- 16:48 What people get wrong with multi-touch attribution
- 25:44 Dealing with the challenge of the iOS14 update
- 27:50 The role of marketing mix modeling
- 33:51 Validation: Engaging customers after conversion
Quotes
(2:25-2:52) “When you’re thinking about any system, especially a complex system, and you’re given a problem, you need to decide which level of granularity you choose to model and understand that system. So different levels enable different insights, but it’s also a practical thing. If it’s a really complex system it may be too much to understand at the atomic level. What I say is you can’t predict anything at the atomic level because there’s too much going on. And we know this in physics, too.”
(24:23-24:35) “When I come to a website, I don’t care what channel I came through. I don’t think about it consciously. There’s no reason to organize how you measure incrementality based on channels. Channels don’t exist. Customers exist.”