The Pros and Cons of Attribution and Incrementality
May 09, 2019
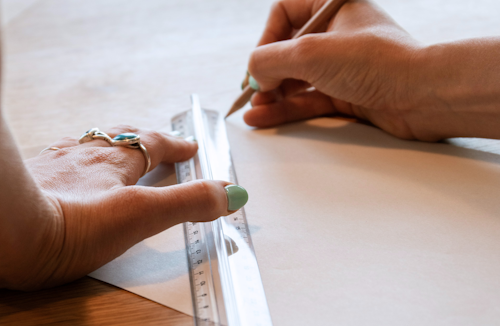
Attribution has been the main method of analyzing campaign performance for several years now. While the concept of attribution is widely used, there are several shortcomings with attribution models, which explains why the topic of incrementality measurement is growing in popularity.
Outline of attribution models
All attribution models have one thing in common: they correlate customer touchpoints with ads (impressions, clicks) and their purchase behavior (conversions).
There are different model concepts like Click Through, View Through, Last Touch, First Touch, Multi-Touch and others. All the models rely on timely correlation of events per user (“first this happened, then that happened for the same user”) to derive the concept of attribution, which in fact is often being perceived as causality (“the user clicked on that ad, so we attribute this revenue to that ad” sounds very similar to “that ad caused that revenue”).
However, as we know, there is usually a large variety of factors influencing the purchase decision including brand equity, word of mouth, offline media campaigns, and more, making it questionable to attribute all the revenue to that one ad the user clicked on. Unfortunately, no attribution models can account for those factors. This is where incrementality measurement can help.
Outline of incrementality measurement
The concept of incrementality measurement uses a different principle from attribution models.
Rather than relying on the correlation of events, it uses a Randomized Controlled Trial (RCT) to observe differences in behavior (conversion rate, revenue per user, etc) between a ‘treatment group’ that is targeted by ads and a ‘control group’ that is not targeted by ads.
If the groups are truly random and there is a difference between the behavior of the group targeted by ads of certain campaigns to those who were not, this proves true ‘causation’ between the campaign and the change in behavior.
Within incrementality measurement, there are a variety of methodologies which differ mainly in terms of what happens to the control group and how to look at results. The most widely used methodologies nowadays are Intent-to-Treat, PSA/Placebo, and Ghost Ads. In retargeting there is also a closely related methodology to Ghost Ads: Ghost Bids.
All methodologies have different pros and cons taking different trade-offs into account: While Intent-To-Treat is free of cost and is easy to implement on the client side, the data is very noisy and often doesn’t show uplift because of that.
- PSA/Placebo solves the problem of noise but creates cost for the impressions in the control group.
- Ghost Ads are free of cost and have no noise, though only work for user acquisition campaigns and not for retargeting.
- Ghost Bids are free of cost, work well for retargeting have lower noise than ITT, but higher than Ghost Ads.
Attribution
Pros
The main advantages of attribution models are that they are easy to implement and reason about. There are multiple vendors in the market which provide an easy-to-use independent solution (Attribution Providers). A further advantage is that attribution models can work with very little data and can measure the relative performance down to the level of campaigns or creatives.
Cons
One of the main disadvantages of attribution models is that they do not account for ‘immeasurable’ contributions like brand equity, offline marketing, and word of mouth. A further problematic area is the lack of accounting for organic behavior. For example, in user acquisition campaigns which already have strong brand equity (think: install campaigns for Uber in San Francisco). This is an even bigger problem in retargeting where there is potentially strong organic behavior from users who already installed an app, which is why applying attribution in retargeting is often missing the point of measuring the actual value of retargeting campaigns.
Incrementality
Pros
The main advantage of incrementality is the objective measurement of the absolute contribution of a campaign to an increase in revenue or conversions. The scientifically developed method of RCT proves actual causation between ad spend and incremental revenues. The incrementality methodology also accounts for organic behavior and any other marketing activities, since both the control and treatment groups are being equally affected by those.
Cons
The disadvantages of incrementality lie in the complexity of this methodology. On the surface, incrementality measurement might seem like an easy concept: randomly split the population, only show ads to one group, then observe the results. Though this is just the tip of an iceberg - to apply it successfully one requires much more detailed knowledge in the areas of different methodologies, statistics, parameters affecting noise, analysis framework, typical biases, and flaws. Another problem with incrementality is that it requires much more data (sample size in unique users) to determine statistically significant results. Therefore it is difficult to apply it on a granular level: per segment/per campaign results.
Analyzing results: attribution and incrementality are independent concepts
While both attribution and incrementality can be meaningful measurements for the same campaign, it is important to understand that those are completely independent (orthogonal) concepts and looking at results need to be independent as well. Both concepts should specifically not be mixed, i.e. looking at attributed conversions/revenues for the treatment group in the incremental results.
While the attributed view of results will include attributed revenue to ‘clickers’ (with Click-Through-Attribution), incremental measurements will observe all revenues/conversions of the respective group (treatment/control) regardless of click or view.
Another difference is that the attributed results will usually include some attribution windows, while the incrementality measurement does not need/use that concept - since there are no clicks/views for the control group part of the population. Instead, the incremental measurement observes all behavior of the two groups for a certain period of time (usually the campaign runtime and the delayed conversion grace period).
A less intuitive concept in incrementality results is one of ‘exposed users’. Intuitively one wants to look at revenue/conversions of users exposed to ads’ vs ‘users not exposed to ads’. This is only possible in certain methodologies, where the treatment group contains ‘only exposed’ users and the control group only the ‘would have been exposed’ users. Such methodologies are PSA/Placebo and Ghost Ads, which have the information about ‘which users would have been exposed in the control group’.
The methodologies Intent-to-Treat and Ghost Bids include ‘exposed’ and ‘unexposed’ users in the treatment group (to a different degree) and don’t provide the information which users in the control group ‘would have been exposed’. Therefore in these methodologies, one has to look at the behavior of the total groups (‘exposed and unexposed’ in the treatment group and ‘would have been exposed and would not have been exposed’ in control group). Looking at ‘treatment exposed’ vs ‘all of the control group’ in those methodologies is not possible, because it would suffer from selection bias: the selection process of ‘who is exposed’ in the treatment group is not a random one and is highly affected by mechanisms of targeting, optimization and auction dynamics.
A common counterargument to looking at unexposed users in the treatment group is: “The unexposed users haven’t seen any ads, didn’t create any cost and we shouldn’t look at their conversions/revenues”. This argument is valid with PSA/Placebo and Ghost Ads methodologies, though it doesn’t work for Intent-To-Treat and Ghost Bids due to selection bias. Though it is important to understand that - contrary to attribution - the revenues of the unexposed users are not simply ‘attributed’ to the campaign - they are used as a sum with the revenues of exposed users to then later be compared to the revenues of the control group (potentially including scaling).
If all users in the treatment group were unexposed, there would be no uplift and no difference between the two groups, resulting in no incremental revenue. This illustrates that looking at unexposed users is not a problem but a methodological necessity to avoid biases.
Conclusion
Both attribution and incrementality have their place within an effective mobile marketing strategy. The key is to understand their separate functions and be aware of the limitations of each. With this in place, you can build an accurate picture of how each of your campaigns are performing and what actual value it’s are driving.