Incrementality Tests 101: Intent-to-treat, PSA and Ghost Bids
September 23, 2019
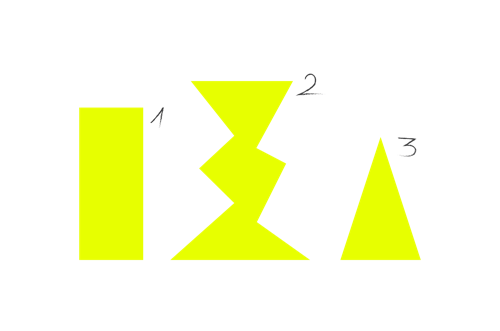
« When a measure becomes a target, it ceases to be a good measure. »
Goodhart's Law
Economist Charles Goodhart nailed it in his adage. In a popular application of this law, two workers are asked to create nails. One’s performance is measured on the number of nails they made while the other is judged on the weight of the nails made. What was the result? A thousand nails for the first worker, and only a few heavy ones for the second. Their performance incentives are tied directly to their goals.
In mobile marketing, we observe similar issues, depending on each advertiser’s goals. With shifting industry goals around performance KPIs come new attribution models, and more often than not problems along with them (fraud, cannibalization, last-click stealing, and so on). As seen in Goodhart’s law, wrong metrics can lead to wrong incentives, which ultimately lead to advertisers and other industry professionals to the question “how much of the total revenue is truly caused by my advertising spend?”
Understanding the relationship between ad spend and revenue is the only way to mitigate the risk of ineffective spending and budget. While attribution models are easy to implement and most commonly used, these models don’t scientifically prove causation. Cum hoc ergo propter hoc, or in other words “correlation does not imply causation”.
Applying Medical Methodologies in Advertising
Enter Randomized Control Trials (RCT). RCT is a widely used concept for multiple scientific practices. In clinical trials, RCT shows whether the new drug, device, treatment and such truly have an effect on the user (positive, negative, or none).
“Randomized controlled trials (RCTs) are widely taken as the gold standard for establishing causal conclusions. Ideally conducted they ensure that the treatment ‘causes’ the outcome—in the experiment.“
- Nancy Cartwright, "What are randomized control trials good for?"
In retargeting, the ad is given in place of medication
RCT is done by splitting the subjects into two groups and testing one with the new medication. One group, namely the test group, is given new treatment, while the control group remains untreated. Any observed change within the test group is noted as an effect of the treatment. As RCT methodologies scientifically prove causality, using these concepts for incremental testing gives an unbiased reflection on the efficacy of mobile remarketing.
In retargeting, the target audience will also be split into two groups, where the test group receives advertising campaigns, and the control group does not. In incrementality measurement, there are a few methodologies that differ mainly in terms of what happens to the control group and how to look at the results.
Different Methodologies Applied in Advertising
Intent-to-Treat (ITT)
No cost and easy to implement but with noisy data
Let’s begin with the intent-to-treat methodology. In all cases, the ITT method does not show any ads to users within the control group. Also known as the ‘holdout test’, this approach measures uplift by:
1. Complete comparison: This method compares the behavior of all users in both groups (both exposed and unexposed to ads in the test group with the behavior of the control group).
This method is the correct way of applying Intent-to-treat as it answers the question: “How much more are the users who are targeted by my ads spending compared to a control group?”
While correct, this method creates the problem of noisy data (more below on noise).
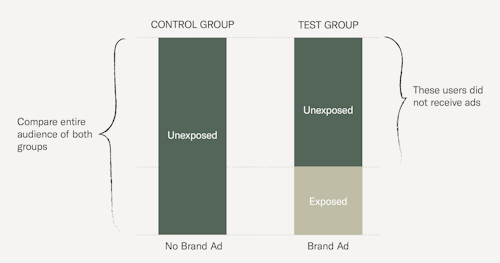
2. Partial comparison (naive method): This method compares the behavior of only exposed users with the probable behavior of the control group.
In an effort to reduce the noise from all unexposed test group users, this second approach to ITT is a common mistake that leads to selection bias because of the availability of supply, lost auctions, and impression rendering.
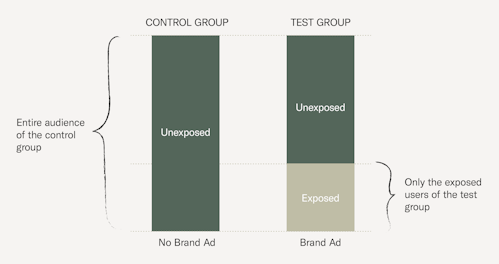
The Intent-to-treat methodology for incrementality measurement is widely used because of its ease of implementation on the client-side (i.e. BI team or data science) and won’t need to be integrated into the ad delivery system of the advertising partner.
However, there are situations where only a small fraction of the users in the treatment group will be exposed to the ads. This can happen due to the low availability of the targeted audience on the supply channel or low win rate on programmatic auctions. Consequently, including a potentially large proportion of unexposed users in the treatment group makes the measurement noisy.
Noise in data
Noise, a.k.a. meaningless information stems from the unexposed population of the test group. Noise often leads to unsuccessful uplift tests with no statistical significance nor uplift. This comes from the fluctuations in the unexposed group’s behavior which overshadow the uplift stemming from a small exposed population.
The problem with 'noise'
Have a look at the graphic below:
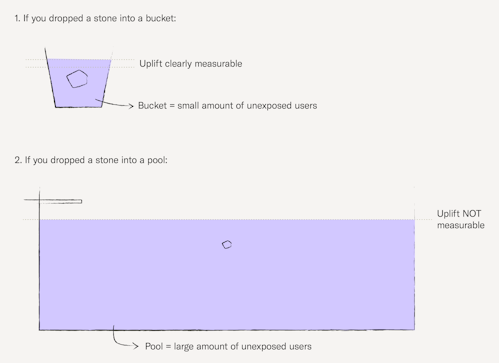
If we put the same rock in a bucket of water and into a swimming pool, where would it be easier to visually measure the change in the water levels? Easy answer - the bucket. The aim is to see the difference, and in uplift measurement the swimming pool represents a large amount of unexposed users, making it hard to detect the impact of a rock.
Public Service Announcement (PSA) or Placebo Ads
The zero noise but costly and unsustainable alternative
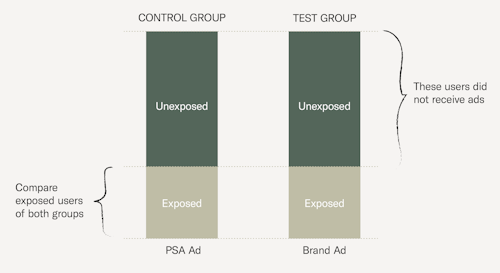
In the ‘PSA/Placebo’ methodology, the control group receives a real ad. The idea is to serve the control group public service announcement (PSA) ads - ads that help raise social awareness like red cross banners or don’t drink and drive ads.
By serving real ads, we obtain the information on which users within the control group would have been exposed, allowing us to exclude unexposed users from the measurement. This reduces noise to zero.
While PSA ads are easy to use in self-service platforms and are a great workaround for the noise problems drawn from Intent-to-treat testing - in fact, PSA uplift testing generates no noise at all - this method is costly and unsustainable. Marketers would have to provide a portion of their budget to pay for the control group impressions, consequently reducing their profit margin. Since incrementality testing and measurement are most effective when ran continuously (not a one-time test), allocating budget for PSA ads isn’t really an appealing long-term strategy.
PSA ads can also be inaccurate if implemented incorrectly. For example, when running two different campaigns in a smart ad delivery system, the system is likely to optimize the delivery of the two campaigns differently because the ads are inherently different:
- "[The system] will show ads to the types of users that are most likely to click. And the users who choose to click on an ad for sporting goods or apparel are likely to be quite different from those who click on an ad for a charity - leading to a comparison between “apples and oranges.” Hence, such PSA testing can lead to wrong results ranging from overly optimistic to falsely negative.” - Think with Google
It would also be an assumption that the control group behavior is completely comparable to the test group. PSA testing ignores the chance that the same user might react more strongly to don’t drink and drive ads than say, unlock-a-new-character-in-the-next-level-play-again type of ad. The definitions of the groups are ultimately distorted, making it impossible to have a real comparison.
Ghost Ads
The best of both ITT and PSA, but is more suitable for User Acquisition
As discussed in several publications, the Ghost Ads concept offers the most advantages in incrementality measurements. This includes low noise and lowest selection bias with the further advantage of being free of cost for the advertiser - the control group is not exposed to your ads, therefore incurring no additional costs (as opposed to the PSA approach).
Ghost ads offer an improvement of the PSA concept by removing the costs for the advertiser. The control group users are shown another ad ran by another advertiser on the platform, therefore removing the cost for clicks and impressions. The control group behavior is then marked with a “ghost impression”, giving us the information on which control group users would have been exposed.
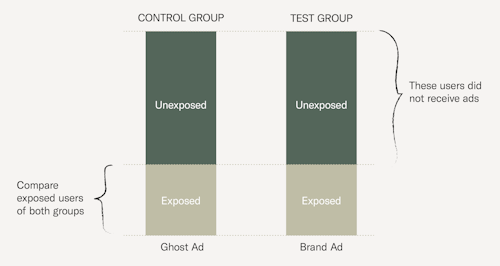
While Ghost Ads are precise and accurate, the method doesn’t work well for retargeting. Ghost ads require a second interested party for the user in question. This is easily done within the user acquisition space because a user is potentially relevant/interesting for multiple advertisers. In retargeting, it is often the case that there is only one party interested in the user at hand since retargeting campaigns usually target very specific and narrow user segments.
Ghost Bids
A more precise and cost-free incrementality testing method for app retargeting
Similar to Ghost Ads, the goal is to remove as much noise and unexposed users as possible. We used the concept behind Ghost Ads as the foundation for our continuous uplift tracking product, though our implementation differs from the original implementation in several points to better adapt it for retargeting, therefore calling our approach ‘Ghost Bids’.
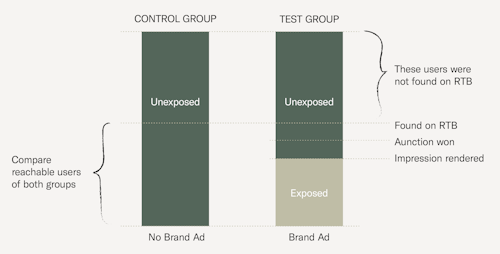
All users that are not found on RTB from both groups are removed, thus significantly reducing noise.
To be able to measure incrementality, we track revenue and conversions for all ‘reachable users’ - those who fall into the target segment and are seen on RTB ad exchanges, and for which we can place a bid. A bid is placed as usual for the test group, whereas the control group is tracked with “Ghost Bids” (A bid could have been placed, hence the name ‘ghost bid’). Users who fall into the target segment but are not seen on ad exchanges are not part of the test.
Noise is therefore significantly reduced compared to the Intent-To-Treat method since users who are not available on RTB supply are not corrupting the measurement (their behavior is irrelevant for our campaigns).
This means we have two groups of users in the treatment group: exposed (seen at least one impression) and unexposed (we didn’t win any auctions for this user or none of the impressions rendered).
Similarly, in the control group, there will be users who ‘would have been exposed’ and ‘would not have been exposed’. Although the unexposed share of users creates additional noise in the test, there is no easy way to predict which users in the control group would have been exposed without adding any potential bias to the test.
Wrapping Up: Incrementality Measurement
- Understanding the concept of incrementality testing is the first step in having a more scientifically-based figure of your ROI. Knowing the different methodologies along with their pros and cons is the next step in making informed decisions.
- Having the right incrementality measurement tools and methods provide the right incentives for both advertisers and vendors. Incrementality as a strategy will eventually make mobile retargeting a more transparent, measureable, and less fraudulent channel in the long run.
For further readings on Incrementality Measurement, we’ve compiled a few scientific papers in another blog post.
Get the latest app marketing insights
Join our newsletter